Executive Question and Answer Session: Data Science In Malaysia
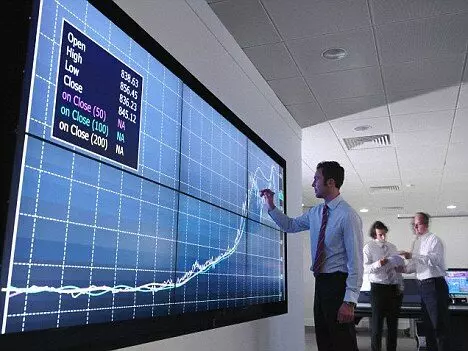
After decades of realising the importance of unstructured data and the massive amounts of unstructured data collected, organisations continue to struggle with incorporating it into their analytics. What difficulties do they face?
Unstructured data is difficult to store in a column-row database, such as a spreadsheet. Because unstructured data comes in a variety of media, including movies, photos, and phone calls, it lacks a standardised framework for analysis. Even when organisations convert unstructured data to structured data, they must evaluate it and accomplish their goals using artificial intelligence. Most businesses lack the funding or time necessary to construct such a product.
Another issue is that the volume of unstructured data is growing at an alarming rate, at approximately 55-65 percent every year. Regardless of whether organisations manage data online (in the cloud) or offline (on a local server), the expense of AI may be prohibitive.
How far have we come in the previous decade in terms of utilising unstructured data? data science in malaysia
Ten years ago, organised data ruled the world. After 2012, however, as sensors grew more affordable, mobile phones progressively evolved into smartphones, and cameras were add to facilitate shooting. This resulted in the generation of a vast volume of unstructured data, and organisations entered unfamiliar ground, slowing their growth. Several impediments to advancement in this field include the following:
Complexity: Unlike structured data, which can be analyse intuitively, unstructured data requires additional processing and analysis, which is typically best accomplished via artificial intelligence. Algorithms for machine learning classify and identify the content. However, identifying high-quality data within the data collection is difficult due to the volume and complexity of unstructured data — this has been a source of frustration for developer teams and a significant problem for already complicated data architectures.
While the organisation acknowledges the importance of unstructured data, the expense of utilising it might be an impediment. Costs associated with organisational infrastructure, human resources, and time might obstruct the installation and development of artificial intelligence and the data it analyses.
What progress has been made in the last decade in analysing unstructured data – or has there been none at all? Are corporations still unable to examine it due to a lack of tools?
As previously said, unstructured data has played a minor role in the last decade due to its complexity and rapid growth. Without a fitted AI training model, enterprises would be unable to separate high-quality data from existing data sets. However, a wonderful illustration of advancement is Data Version Control (DVC), an open-source version control system for machine learning projects that was introduced on GitHub in 2017. DVC was created to facilitate the sharing and reproducibility of machine learning models. It is optimise for huge files, data sets, machine learning models, and measurements, in addition to code.
What tips or advice would you give to businesses looking to begin studying unstructured data? How are they to begin? data science in malaysia
We recommend that organisations develop a comprehensive plan before beginning to analyse unstructured data. Due to the rapid growth of unstructured data, organisations must carefully examine the following before collecting data: where to store the data (in the cloud or on-premises); how to identify high-quality data; how to construct the training model and iterate using newly received data, and so on. Additionally, artificial intelligence practitioners can assist organisations in defining the queries (and their associated answers) prior to collecting and analysing unstructured data.
Data science in Malaysia is getting more important and they should take the data science course Malaysia to catch up that knowledge for advanced career planning.
What types of insights are revealed by unstructured data?
Unstructured data can be mine for valuable information, and its worth varies by industry. For instance, we recently teamed with a supply chain logistics company to provide intelligent logistics. The startup offers artificial intelligence monitoring services aimed at simplifying warehouse operations. The client then uses computer vision to identify and categorise the inventory based on the data collected. Additionally, this data enables more accurate forecasting and planning of logistics, as well as increases production productivity, accuracy, and efficiency.
Another instance is find in the car business. Users’ experiences with connected cars may provide direct feedback on the product. Such unstructured data can be handle and evaluated for purposes such as product development, automotive development, quality improvement, production, and, of course, consumer pleasure.
How is Graviti facilitating the usage of unstructured data?
Graviti’s mission is to build the world’s first data platform that enables enterprises to interact with massive amounts of unstructured data to fuel new artificial intelligence applications. This technology reduces the bother and enables developers to collaborate on managing vast amounts of unstructured data.
Although the majority of available data in AI development is of low quality and unstructured, development teams typically spend more than half of their time discovering, augmenting, or cleansing unstructured data, and that’s only the beginning. Graviti frees developers from data management tasks and gives them more time to study unstructured data and train machine learning models. We assist developers in three areas: data discovery, iterative data processing, and workflow automation.